Specialized AI Pipelines for Parts & Service Data
Predii automates the cleansing and tagging of large, unstructured datasets, transforming repair and service data into actionable insights.
Predii’s Specialized AI Pipelines are designed to efficiently process and structure large volumes of unstructured automotive data. This includes repair logs, parts catalogs, service histories, and technical bulletins. The pipeline automates the critical tasks of data ingestion, cleansing, and tagging, ensuring that raw data is transformed into a usable, structured format with minimal manual effort.
​​
Automated Ingestion & Preprocessing
Data Ingestion: Automated ingestion of unstructured data from a variety of sources, including repair logs, manuals, service bulletins, and ERP systems. Predii handles multiple data formats and leverages NLP (Natural Language Processing) for semantic understanding.
Pre-Processing: Natural Language Processing (NLP) extracts meaningful text from documents. This includes tokenization, stop-word removal, and data normalization to standardize formats and ensure consistency in the text before feature extraction.
Feature Extraction & Data Standardization
Automated Feature Extraction: Specialized machine learning models identify key features from the text, such as symptoms, Diagnostic Trouble Codes (DTCs), parts, labor operations, and related service activities.
​
Data Normalization: Data is transformed into standardized formats, ensuring consistency in terminology (e.g., part numbers, fault codes, and repair procedures).
Automated Tagging: Each piece of data is tagged with relevant labels based on the predefined ontology. ​​
​
Ontology & Taxonomy Alignment
Ontology: Defines the structure of automotive-specific features, specifying entities (e.g., parts, failure codes, repair procedures) and their relationships.
​
Taxonomy: Ensures uniform classification and terminology across diverse data sources. This step helps align all extracted features to a common set of definitions and categorizations.
​
This serves as an automotive-specific knowledge graph for parts & service data.
Data Clustering & Aggregation
​Data Aggregation: Aggregates features from multiple sources and compiles related data points into a unified dataset, enabling more comprehensive analysis of i.e. top symptoms, top repairs, top parts. Helps understand complex multi-line repair jobs.
​
Clustering: Consolidates data around features. Clustering allows grouping and leveraging of data, enables identification of repair patterns and related repair jobs, helps detect trends, and detects correlations.
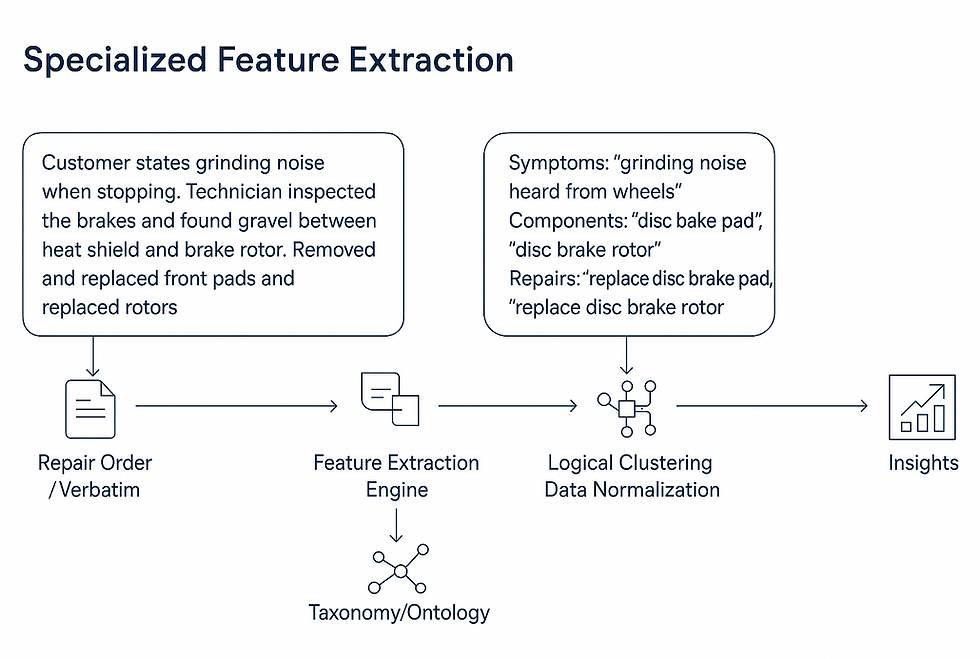